Causal Assumptions
Assumptions
The followings are causal assumptions (those not explained inline will be explained below):
- Stable Unit Treatment Value Assumptions (SUTVA)
- Consistency: what you observed (under one of the treatment) is equal to the true underlying potentials
- Ignorability
- Positivity: for all covariates , because if some group is never treated, , then we cannot learn the effect
Assumptions can be about observed outcome , observed treatment , and covariates .
Stable Unit Treatment Value Assumptions (SUTVA)
No interference: units of interest do not interfere with each other. In most settings units are people. Situations that these are violated:
- Contagion: The sickness of one people affect the other; or Vaccine
- Behavioral Study: when people’s behaviors are interacting with others
One version of treatment
SUTVA allows us to write potential outcome for the ith person in terms of only that person’s treatment. This simplifies the problem.
Ignorability
This is (2). I will elaborate it more intuitively here. They can be ages, gender, places living in, as long as they affect both the treatment and outcomes. And this assumptions is saying: Among people with the same values of , we can think of treatment are randomly are assigned. The following example helps:
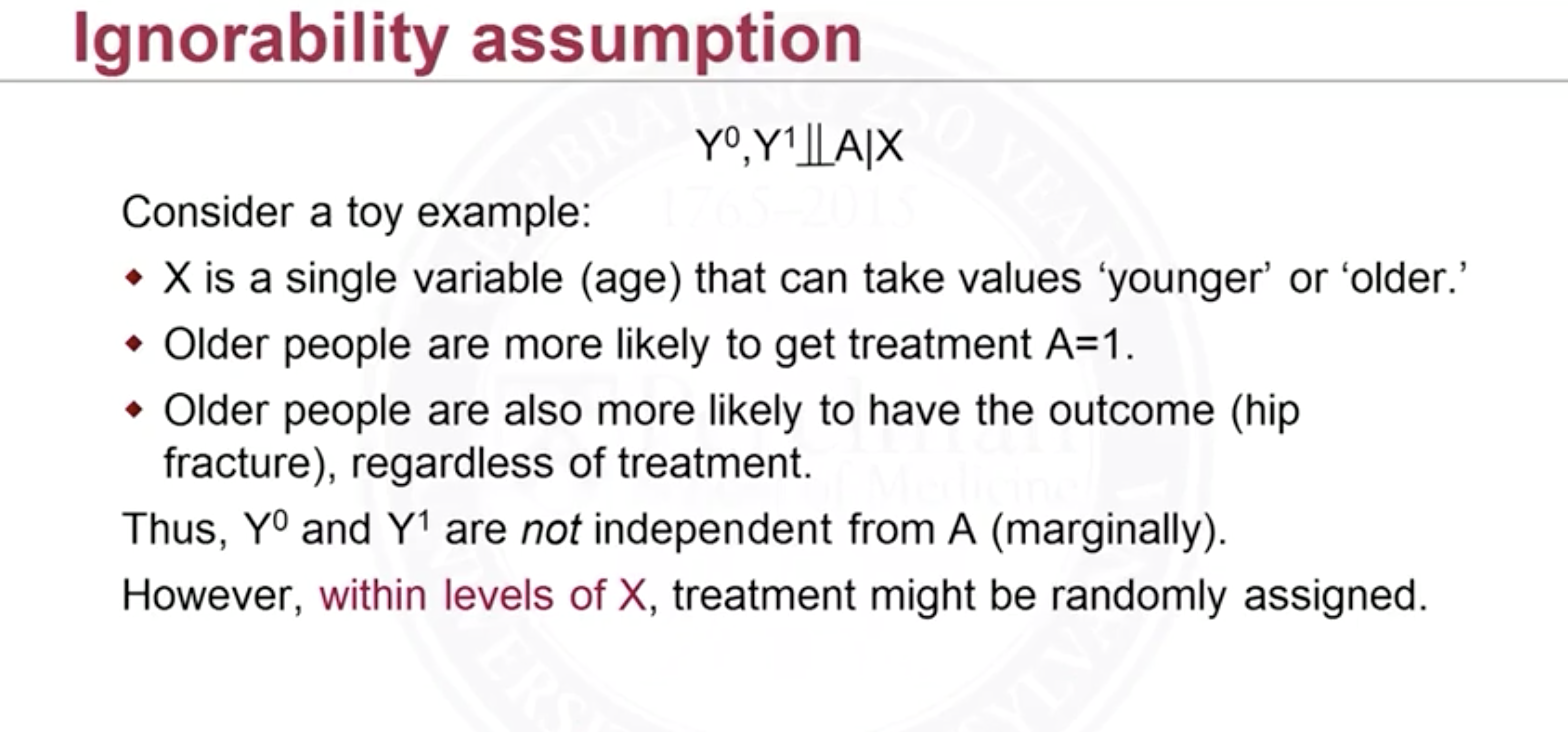
And we need to figure it out what we need to collect in order to make that assumption satisfied.
Standardization
Standardization is a way of dealing with confounders. We use the law of total expectation to marginalize out the covariates: If we want marginal causal effect, we can average over all , The crucial caveat is that our $P(\mathbf X=\mathbf x^{(i)})=\frac{I(X=x^{(i)})}{N}$ is approximated by the sample. But even when $\mathbf X$ is a scalar $X$, if it’s continuous, we won’t have all of its values and it’s possible there’s some values we don’t have. This become more severe if $\mathbf X$ is vector. We won’t have a good estimate for $P$. We use many techniques such as matching to deal with this problem.